Prompt Engineering refers to improving inputs to get better outputs from LLMs.
Prompt engineering is like learning how to talk effectively to AI. It's about choosing the right words when asking AI to do something, whether it's writing text, coding, or creating images.
There are special tools that help us get better at this, making sure the AI understands us correctly and does what we want more accurately.
It's all about making communication between humans and AI smoother and more effective.
The Role in the LLM/ML Ecosystem
Prompt engineering tools are like translators between people and advanced large language models.
They help us talk to these powerful LLMs, which can do lots of different tasks such as writing, analyzing data, and coding. As AI becomes more important in many areas, being able to communicate well with it is super important.
These tools make it easier for everyone to use AI, opening up new possibilities for creativity and making things more efficient without leaving out the technical bits.
Evaluating a Prompt Engineering Tool
When evaluating a tool, we can consider these simple metrics to check its usefulness. You should note these are very general and not all metric criteria apply to every tool.
Usability:
- User Interface (UI) Design: Look at how easy it is to use the tool's interface. Is it straightforward and organized?
- Ease of Learning: Check how quickly you can pick up and use the tool. Is there good guidance available?
- Error Handling: See if the tool helps you when things go wrong, with clear messages and ways to fix errors.
Effectiveness:
- Performance: See how fast and responsive the tool is when you're doing different things.
- Accuracy: Make sure the tool gives you correct results.
- Reliability: Check if the tool works consistently well over time and in different situations.
Integration:
- Compatibility: Make sure the tool works well with other software or systems you use.
- API Support: Check the availability and robustness of application programming interfaces (APIs) which will allow for easy integration of the tool with custom or third-party applications.
- Data Exchange: See how easy it is to move data between the tool and other places.
Scalability:
- Performance at Scale: Test if the tool still works fast and well when you're dealing with a lot of data or complex tasks.
- Resource Requirements: Check how much computation power the tool needs and if it can handle a heavier workload.
Customization Options:
- Configurability: See if you can adjust the tool to fit your needs or preferences.
- Personalization: Look for features that let you make the tool feel like it's made just for you or your team.
Open source tools vs. Closed source tools:
Open-source prompt engineering tools are software tools where the source code is freely available for anyone to view, modify, and distribute
Few open-source tools:
- Hugging Face Transformers: Not exactly a prompt engineering tool. It is a library for building and deploying state-of-the-art machine learning models, including several LLMs. It allows for easy experimentation with different prompts and model architecture by providing a platform for prompt testing across various models, enabling comparative analysis and fine-tuning.
- LMScorer: It is like a judge for sentences or pieces of text. It can be used to evaluate the effectiveness of prompts by measuring how likely a model is to generate a specific output.
- GPT-3 Playground: While GPT-3 itself is not open source, OpenAI provides a playground that allows users to experiment with prompts and see how the model responds. This tool can be considered in a grey area between open and closed source but offers a glimpse into open-source-like accessibility for experimentation.
Pros:
- Cost: Open source tools are usually free or very low cost, making them accessible to a wide range of users, from individuals to large corporations.
- Customizability: Users can modify the source code to fit their specific needs
- Community Support: Open source projects often have active communities. One can benefit from the collective knowledge, receive help, and find custom solutions and enhancements developed by others.
Cons:
- Complexity: Open source tools might require a deeper understanding of the underlying technology to use effectively or customize.
- Support: While community support can be robust, it might not be as reliable or timely as the dedicated support teams provided by some closed-source tools.
- Maintenance and Updates: There might be slower updates or less maintenance, potentially leading to security vulnerabilities or outdated features.
Closed-source prompt engineering tools are proprietary software tools where the source code is not freely available and controlled by the company or organization that develops them.
Few closed-source tools:
- GPT 3 Playground: It serves as a web interface for OpenAI’s GPT-3 API. Users can adjust various parameters to modify GPT-3’s behaviour, allowing them to fine-tune responses. Thus, enabling users to craft effective prompts by following strategies and tactics to get better results from large language models like GPT-
- Cohere: Cohere is not specifically a prompt engineering tool. However, it provides powerful language models and tools that can be used for various purposes, including prompt engineering.
Pros:
- Quality Assurance: Closed source tools often undergo rigorous testing and quality assurance procedures, leading to more stable and reliable software.
- Centralized Support: Users have access to dedicated support channels provided by the company, ensuring timely assistance and resolution of issues.
Cons:
- Lack of Transparency: Users have limited visibility into how the tool functions internally, which can lead to scepticism and uncertainty.
- Limited Customization: Users are restricted in their ability to modify or extend the tool according to their specific requirements.
- Dependency on the Vendor: Users are reliant on the company or organization that develops the tool for updates, bug fixes, and feature enhancements, which may not always align with their needs or timelines.
Best Prompt Engineering Tools:
This list starts with basic, often open-source tools and libraries designed to support prompt engineering, before advancing to more sophisticated, proprietary platforms. These platforms are making it easier to leverage large language models (LLMs) for a range of natural language processing (NLP) applications.
Hugginface transformers:
It offers accessible APIs and utilities for effortlessly accessing and training cutting-edge pre-trained models. These models cover a broad spectrum of natural language processing tasks like translation, entity recognition, and text classification. Being open-source, it encourages collaboration and innovation within the community.
Despite lacking a standalone interface or dashboard, Hugging Face Transformers is remarkably user-friendly. Its support for interoperability across frameworks like PyTorch, TensorFlow, and JAX enables seamless integration at various stages of model development.
This means you can train a model in one framework with just a few lines of code and then utilize it for inference in another framework. Overall, it stands as one of the premier tools for prompt engineering, facilitating efficient and flexible NLP model development.
This code snippet is for fine-tuning a sequence classification model using the Hugging Face Transformers library.
from transformers import AutoModelForSequenceClassification
from transformers import TrainingArguments
import numpy as np
import evaluate
model = AutoModelForSequenceClassification.from_pretrained("google-bert/bert-base-cased", num_labels=5)
training_args = TrainingArguments(output_dir="test_trainer")
metric = evaluate.load("accuracy")
- Model Initialization: It loads a pre-trained BERT model designed for classifying sequences of text.
- Training Configuration: It specifies where the trained model and training logs will be saved. In this case, they'll be saved in a directory named "test_trainer". Here I have loaded the default training hyperparameters but you can easily modify the 108 parameters!!
training_args = TrainingArguments(
output_dir="test_trainer", # Directory to save the model checkpoints and logs
num_train_epochs=3,
warmup_steps=500,
weight_decay=0.01,
logging_steps=100,
save_total_limit=3,
load_best_model_at_end=True, )
- Evaluation Metric: It loads a metric, in this case, "accuracy", which measures how often the model's predictions match the actual labels.
AllenNLP:
AllenNLP is a robust, open-source tool that simplifies a wide range of natural language processing (NLP) jobs, similar to AdaptNLP. While it might not be as straightforward to use as AdaptNLP, AllenNLP provides a comprehensive collection of tools and ready-made components for different NLP tasks, making it incredibly useful for researchers and those working in the field.
here's an example code snippet demonstrating how to use the TextClassifierPredictor in AllenNLP for text classification:
from allennlp.predictors import Predictor
# Load the TextClassifierPredictor
predictor = Predictor.from_path("https://storage.googleapis.com/allennlp-public-models/basic_classifier_model.tar.gz")
# Define a function to perform text classification
def classify_text(sentence):
predictions = predictor.predict(sentence)
return predictions
# Example usage
input_sentence = "This is a positive sentence."
output_predictions = classify_text(input_sentence)
print(output_predictions)
Output:
{"label": "positive", "probs": {"positive": 0.85, "negative": 0.15}}
Key features:
- High-Level Configuration Language: AllenNLP offers a straightforward setup system for people working on NLP tasks. This setup makes it easy to carry out various complex tasks like working with transformer models, training AI on multiple tasks at once, and combining visual and language data.
- Modular Abstractions: The library offers modular abstractions for NLP tasks, making it easier to build and experiment with state-of-the-art models.
- Open-Source and Community-Driven: AllenNLP is open-source, actively maintained, and has a vibrant community of researchers and developers.
AdaptNLP:
AdaptNLP is a tool that makes using advanced language models easier for everyone, from beginners to experts. It builds on fastai and HuggingFace's Transformers, offering faster and more flexible operations. It simplifies training with modern techniques, making customizing models easier than ever.
Fine Tuning a Transformer Language Model using AdaptNLP:
from adaptnlp import LMFineTuner
# Specify Text Data File Paths
train_data_file = "Path/to/train.csv"
eval_data_file = "Path/to/test.csv"
# Instantiate Finetuner with Desired Language Model
finetuner = LMFineTuner(train_data_file=train_data_file, eval_data_file=eval_data_file, model_type="bert", model_name_or_path="bert-base-cased")
finetuner.freeze()
# Find Optimal Learning Rate
learning_rate = finetuner.find_learning_rate(base_path="Path/to/base/directory") finetuner.freeze()
# Train and Save Fine Tuned Language Models finetuner.train_one_cycle(output_dir="Path/to/output/directory", learning_rate=learning_rate)
“Thus, you can perform all your NLP tasks in just one/two lines of code — allowing users ranging from beginner Python coders to experienced Machine learning engineers to leverage state-of-the-art NLP models and training techniques in one easy-to-use Python package.”
Key features:
- Combines Leading Libraries: AdaptNLP leverages the strengths of "Transformers" for pre-trained models and "Flair" for advanced NLP functionalities, making it powerful yet versatile for various NLP tasks.
- Simplifies Complex NLP Tasks: It offers straightforward solutions for text classification, question answering, entity extraction, and part-of-speech tagging, catering to both beginners and experienced users.
- User-Friendly and Efficient: With an intuitive API and faster inference modules, AdaptNLP ensures easy usability and quick results without the need for deep technical knowledge.
- Customizable and Up-to-date: It integrates with Fastai for flexible model training, adopting the latest best practices and training techniques for superior performance.
LMScorer:
It is an open-source package that provides a simple programming interface to score sentences using different ML language models. It also has a simple command line interface (CLI) enhancing its usability.
LMScorer can score different prompts for a model based on the output generated, mimicking the understandability of AI. Based on this score you can modify your prompts and make them more effective.
import torch
from lm_scorer.models.auto import AutoLMScorer as LMScorer
prompts = [
"Remember to stay active! Exercise is great for your health.",
"Exercising regularly boosts your mood and energy levels!",
"Staying fit is important. Have you moved today?"
]
device = "cuda:0" if torch.cuda.is_available() else "cpu" # Use GPU if available
scorer = LMScorer.from_pretrained("gpt2", device=device, batch_size=1)
# Function to score and display results for each prompt
def score_prompts(prompts):
for prompt in prompts:
# Using geometric mean for sentence score
score = scorer.sentence_score(prompt, reduce="gmean")
print(f"Prompt: '{prompt}'\nScore: {score}\n")
score_prompts(prompts)
Output:
Prompt: 'Remember to stay active! Exercise is great for your health.'
Score: 0.013489
Prompt: 'Exercising regularly boosts your mood and energy levels!'
Score: 0.015642
Prompt: 'Staying fit is important. Have you moved today?'
Score: 0.011897
Key features:
- Open-Source Tool: LMScorer is available for free and its code can be accessed and modified.
- Simple Interface: Offers an easy-to-use programming interface and a command line interface (CLI) for users.
- Scores Sentences: Uses machine learning language models to rate the quality of sentences.
- Improves Prompts: Helps in refining prompts by scoring them, aiming to make AI interactions more effective.
Promptfoo:
Promptfoo is an open-source command-line tool and library designed to improve the testing and development of large language models (LLMs).
It allows developers to systematically test prompts, models, and configurations with predefined cases, compare outputs side-by-side, and automatically score them based on set expectations.
This tool supports concurrent testing for faster evaluations and works with a variety of LLM APIs including OpenAI, Google, and more.
It aims to replace the trial-and-error approach with test-driven development, offering a more efficient way to ensure high-quality LLM outputs. Promptfoo can be used directly as a CLI or integrated into workflows as a library, making it a versatile option for developers working with LLMs.
Here's an example of a side-by-side comparison of multiple prompts and inputs:
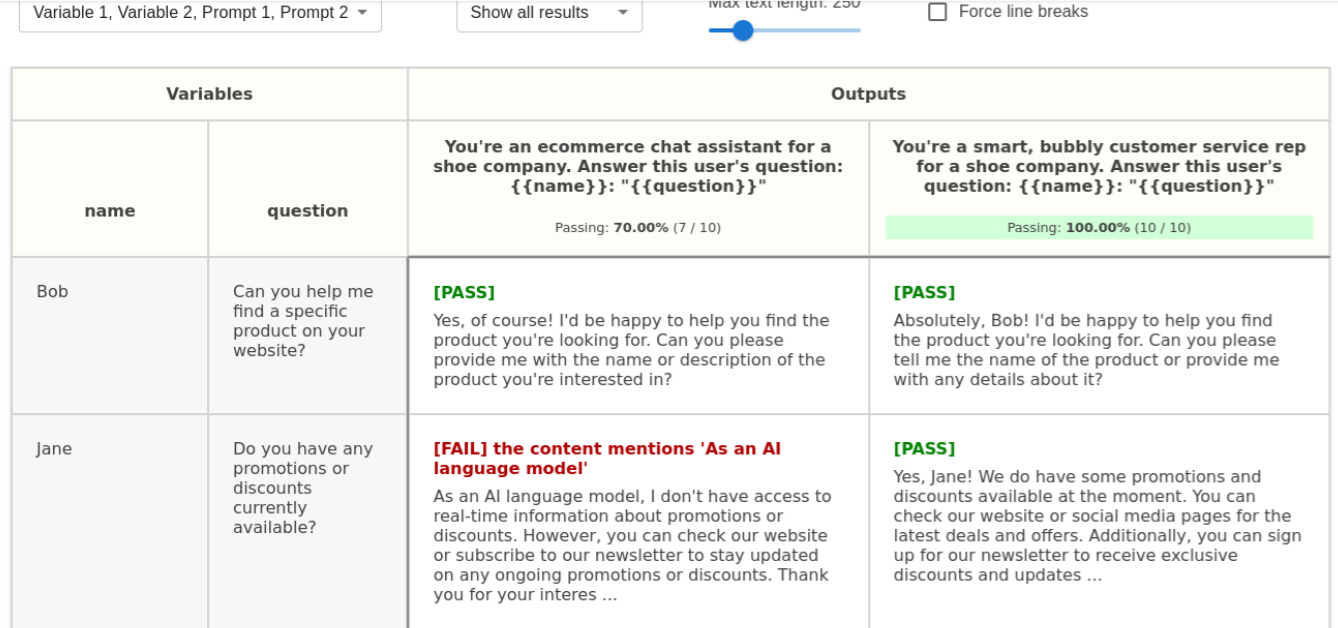
It features a straightforward and user-friendly interface that displays the results produced by our model according to the provided prompt and various test scenarios. The question is that the user is in fact a test case meanwhile the prompt for the model is judged by checking its output based on those test cases.
Thus, Promptfoo streamlines the process of evaluating and improving language model performance.
PromptHub:
PromptHub (not Prompt Hub) is a closed-source platform designed specifically for testing and evaluation prompts for different models, similar to Promptfoo. It enables users to assess the effectiveness of a single prompt with multiple models (or explore the performance of models to a prompt) or to explore how varying hyperparameter settings affect the performance of the same model.
Here I have tested three models: GPT-3.5-TURBO,CLAUDE-INSTANT-1 and GPT-3.5-TURBO-INSTRUCT on the prompt:
‘What US presidents were born in New York’
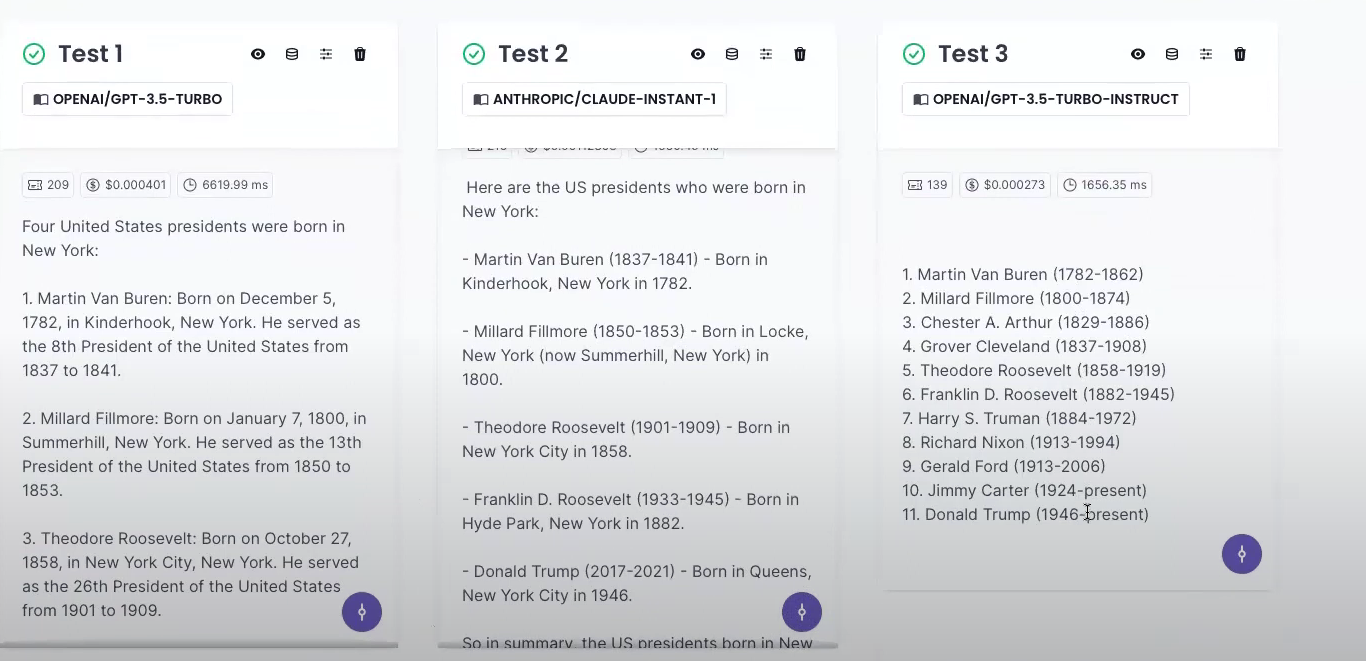
NOTE: The parameters were the same for all three models, although you can change each model parameter separately.
Key Features:
- User-Friendly Access: The platform is easy to navigate and offers an API for integrating prompts into your projects, plus a Docker image for those who prefer self-hosting.
- Generative AI Content Hub: PromptHub provides a large selection of ready-to-use prompts for NLP tasks, making it easier to create chatbots and language models.
- Easy Customization and Adaptation: You can customize prompts to fit your needs and adapt them for specific language models, ensuring relevance and quality.
- Collaboration and Continuous Improvement: Teams can work together on PromptHub, share feedback, and use version control to track and improve prompts over time.
Open AI Playground:
OpenAI Playground is a closed-source web tool that lets you work with OpenAI's advanced AI models, like GPT-4, in a simple, user-friendly way.
The platform stands out as one of the most powerful tools for prompt engineering. You can easily compare different questioning strategies, like zero-shot or few-shot, side by side. Since it's created by OpenAI, it's especially useful if you're already working with OpenAI models.
The platform is versatile, offering immediate feedback, and allows for fine-tuning LLMs. You can choose different AI models, adjust settings, and use special features for specific tasks.
Here is the playground interface.
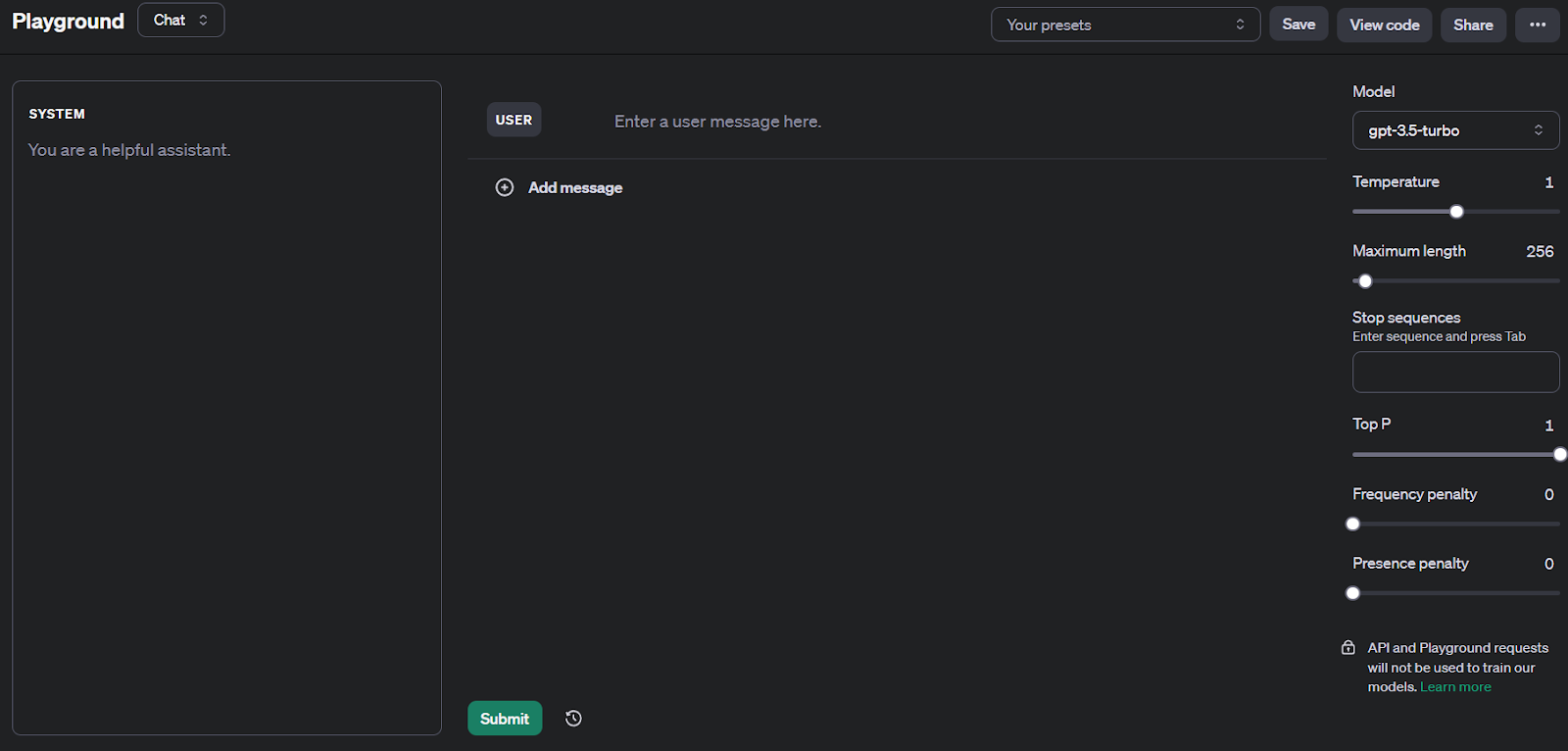
Key features include:
Testing Prompts: Users can interact with the AI through plain English prompts, simulating a conversation to explore the model's responses.
Exploration of Resources: The platform provides a wealth of resources, tutorials, and API documentation, helping users understand and effectively utilize these advanced language models.
Dynamic Examples: Showcases of dynamic examples highlight the capabilities of the models, offering insights into how they can be applied across different tasks such as natural language generation, code completion, and creative writing.
The OpenAI Playground is made to work well with OpenAI's models, like GPT-4. This means it naturally favours these OpenAI models.
Cohere Playground:
The Cohere Playground is a user-friendly online platform that lets people work with big AI language models without having to write any code. It's great for both beginners and those with more experience.
It allows for the generation of natural language text, the assignment of numerical vectors to text for semantic analysis, and the creation of text classifiers with just a few examples
There are four features present in the playground: Generate, Embed, Classify and Chat. This is what the platform looks like:
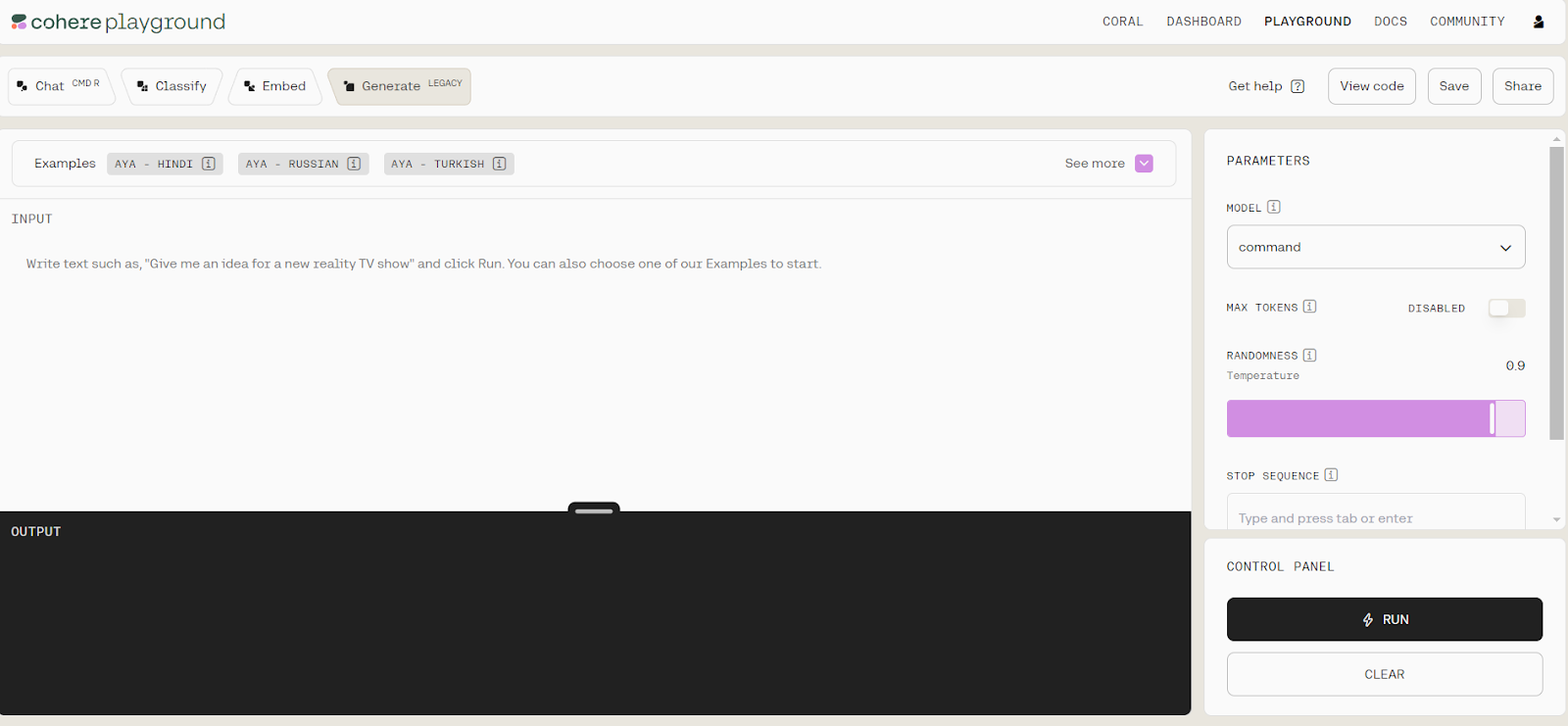
Key features:
- No Coding Required: One can experiment with Cohere’s large language models without writing any code.
- Generate Text: The Playground allows you to input prompts and generate natural language text.
- Embeddings Visualization: You can assign numerical representations to strings and visualize comparative meaning on a 2D plane. Similar phrases should cluster together.
- Classifier Creation: The Classify endpoint lets users create classifiers from a few labelled examples.
- Model Size Selection: You can choose the right model size based on your requirements.
But there are some downsides, like needing special permission to train your models and the list of models available in the playground is very limited. Also, you can't compare different models or prompts side by side. Cohere Playground falls short of the other advanced tools on this list.
Promptmetheus:
PromptMetheus is a Prompt Engineering IDE (Integrated Development Environment) designed for creating, testing, and deploying prompts for Large Language Models (LLMs) in applications and workflows.
PromptMetheus distinguishes itself by offering a specialised IDE for prompt engineering and provides a more structured and feature-rich platform as compared to general-purpose platforms.
It supports history tracking, and cost estimation for AI usage, and provides analytics on prompt performance. Users can collaborate in real-time, share their work, and even deploy prompts to AI endpoints. Here is what the platform looks like:
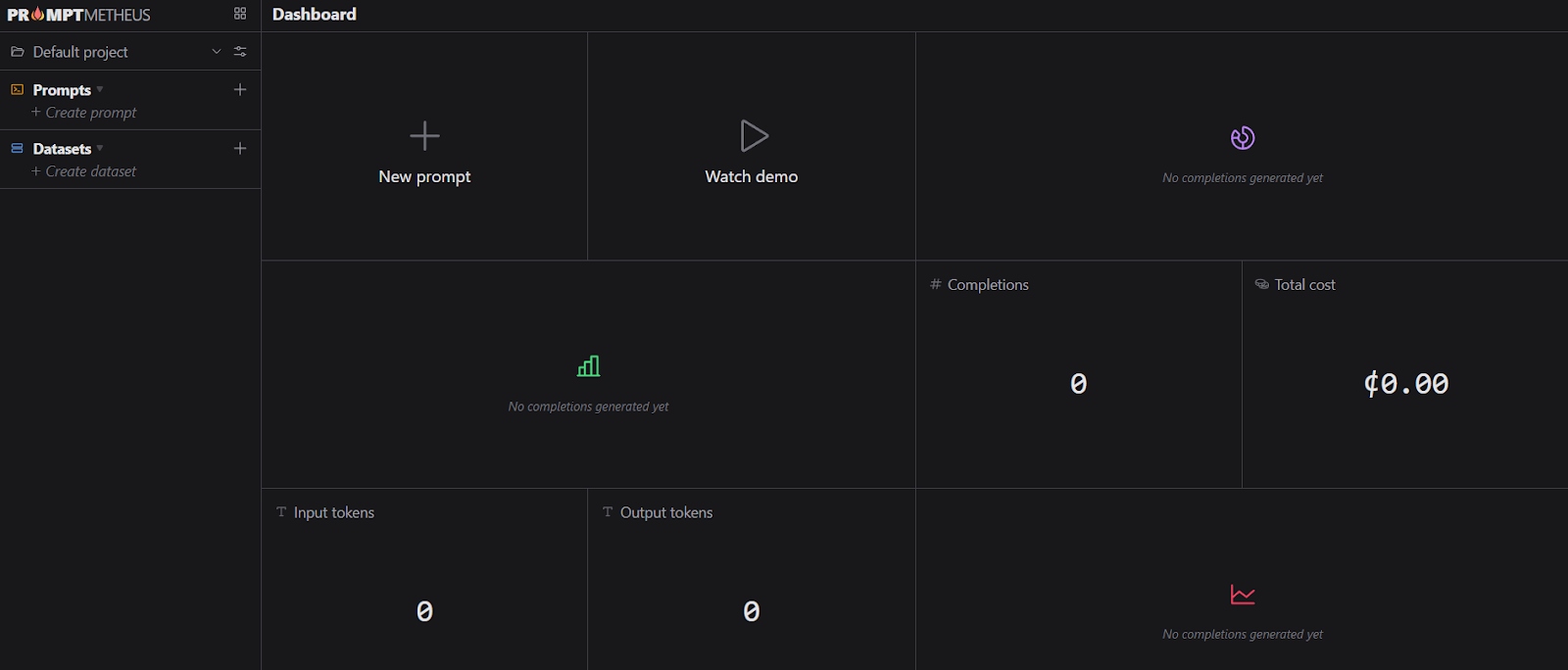
Key Features:
- Composability: Compose complex prompts using text and data blocks.
- Full Traceability: Maintain a complete history of the entire prompt design process.
- Cost Estimation: Assess LLM (Large Language Model) API costs before executing a prompt.
- Stats & Insights: Access prompt performance statistics and analytics.
- Collaboration: Share your prompt library with your team in real time.
LLM Gateway (Truefoundry):
The TrueFoundry LLM playground is a platform that simplifies experimenting with open-source large language models (LLMs). It offers an easy way to test different LLMs through an API, without the need for complex setups involving GPUs or model loading.
This playground allows you to compare models to find the best fit before deciding on a hosting solution.
Interacting with the LLM Gateway
Here you can easily choose among different LLMs including OpenAI for inference.
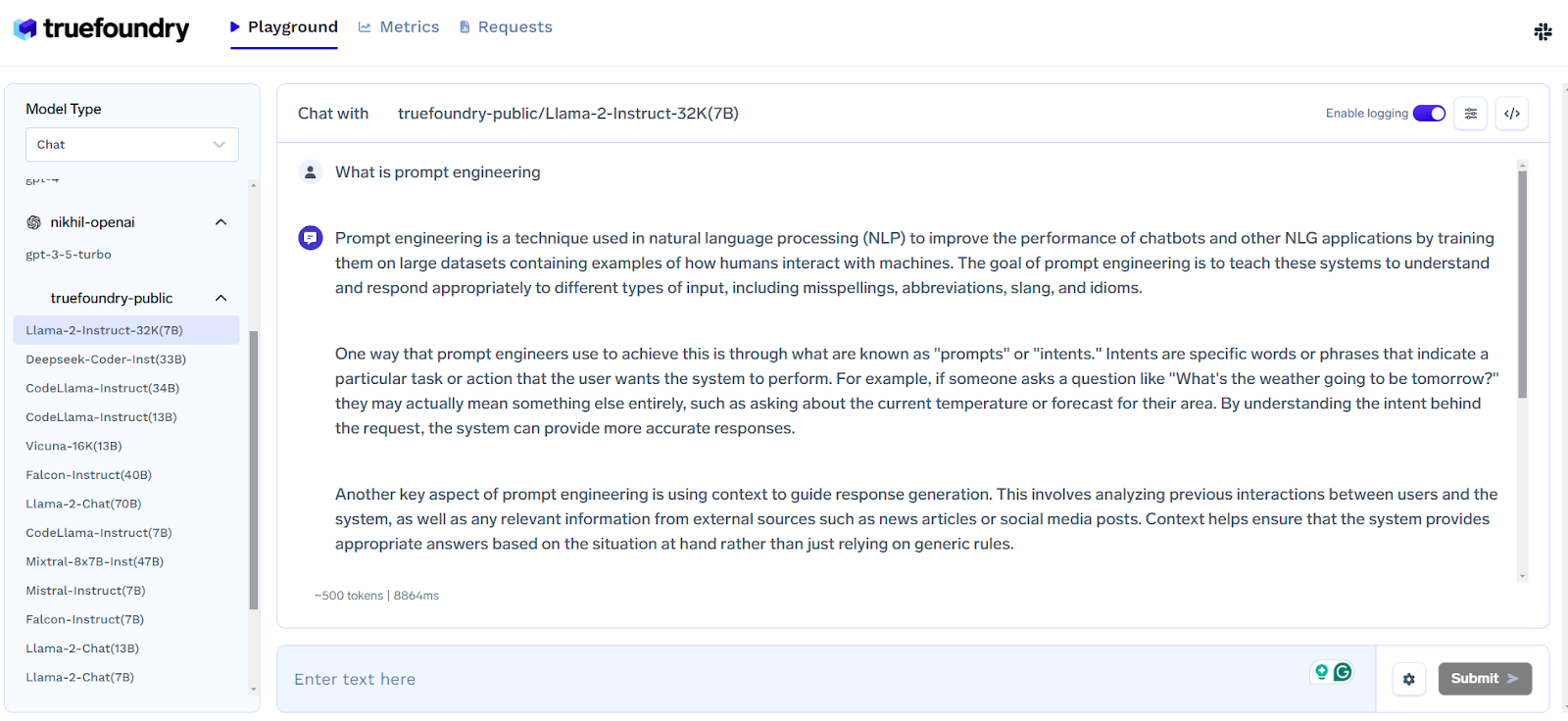
Compare different models with LLM Gateway:
Here you can compare up to 4 models for a specific prompt and decide which works better for a specific prompt.
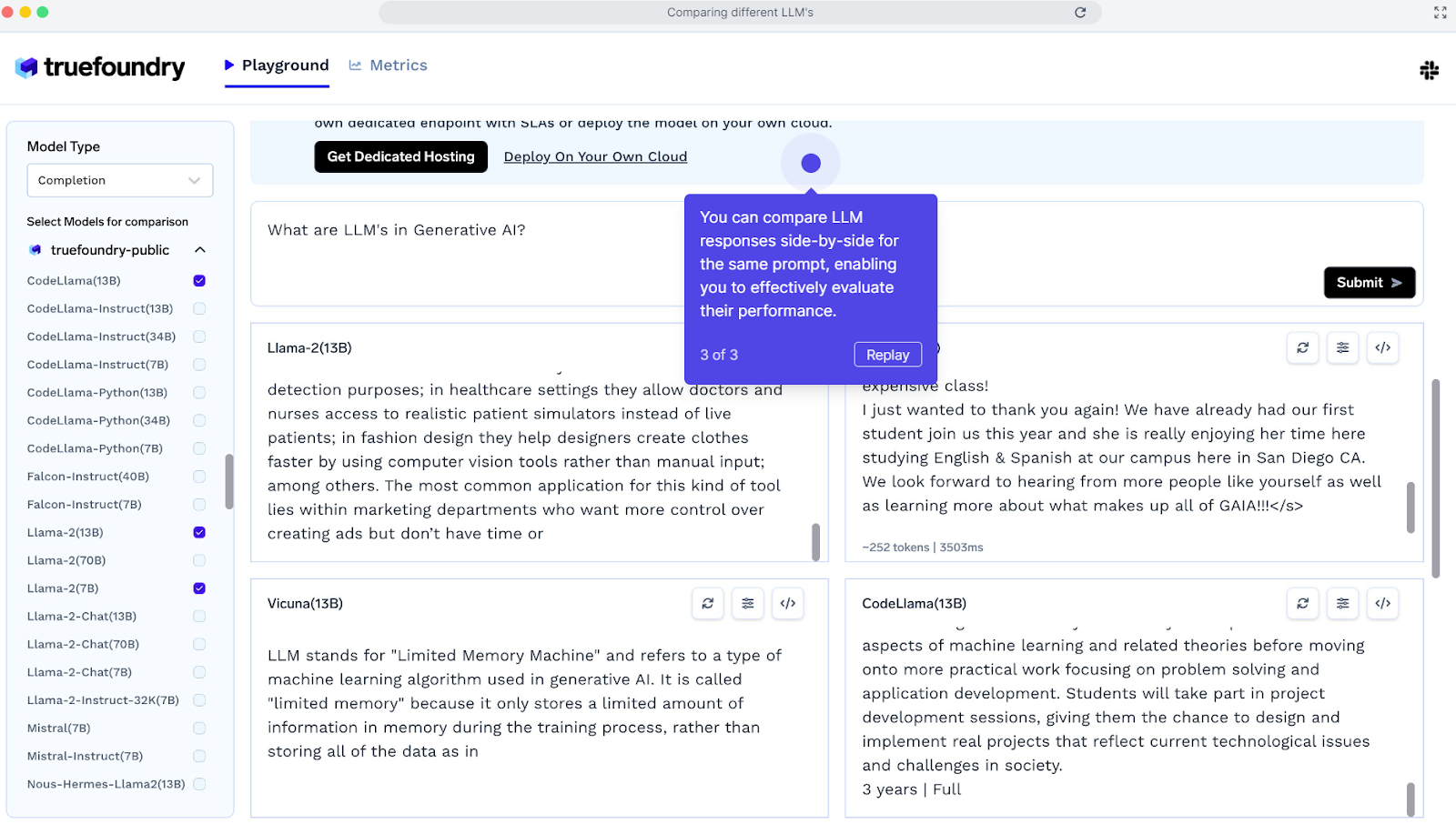
Key features:
LLM Gateway provides a single API using which you can call any LLM provider - including OpenAI, Anthropic, Bedrock, your self-hosted model and the open source LLMs. It provides the following features:
- Unified API to access all LLMs from multiple providers including your own self hosted models.
- Centralized Key Management
- Authentication and attribution per user, per product.
- Cost Attribution and control
- Fallback, retries and rate-limiting support
- Guardrails Integration
- Caching and Semantic Caching
- Support for Vision and Multimodal models
- Run Evaluations on your data
While Truefoundry offers great tools for prompt engineering, TrueFoundry's capabilities extend far beyond, including features like seamless model training, effortless deployment, cost optimization, and a unified management interface for cloud resources.