We are excited to introduce that TrueFoundry has developed a powerful, yet easy to use, solution to Large Language Model (LLM) deployment and fine-tuning through our Model Catalouge
. We aim to help companies self host their open source LLMs on top of Kuberenetes, thus making your inference costs 10x cheaper in 1 click. In this blog we show you how you can deploy a Dolly-v2-3b model and finetune a Pythia-70M model using TrueFoundry.
Would LLMs Change How We Think About MLOps?
TrueFoundry platform has been designed to support Machine Learning and Deep Learning models of all types, from the simplest ones like Logistic Regression to state-of-the-art models like Stable Diffusion. One may think, why does it need to build something new when it comes to Large Language Models?
The sheer size and complexity of these models pose significant challenges when it comes to deploying them in real-world applications. Though the TrueFoundry platform already supported deploying models of all sizes at scale, we realized that there are more optimizations (cost+time) and user experience improvements that we could do for these models.
Large Language Models (LLMs) are here to stay.
Large language models (LLMs), like ChatGPT, have undeniably sparked considerable hype in the field of artificial intelligence.
But having talked to 50+ companies that are already starting to put it in production, the value that it is already creating is immense. We believe that the usage of LLMs is only going to expand as people discover new use cases every day.
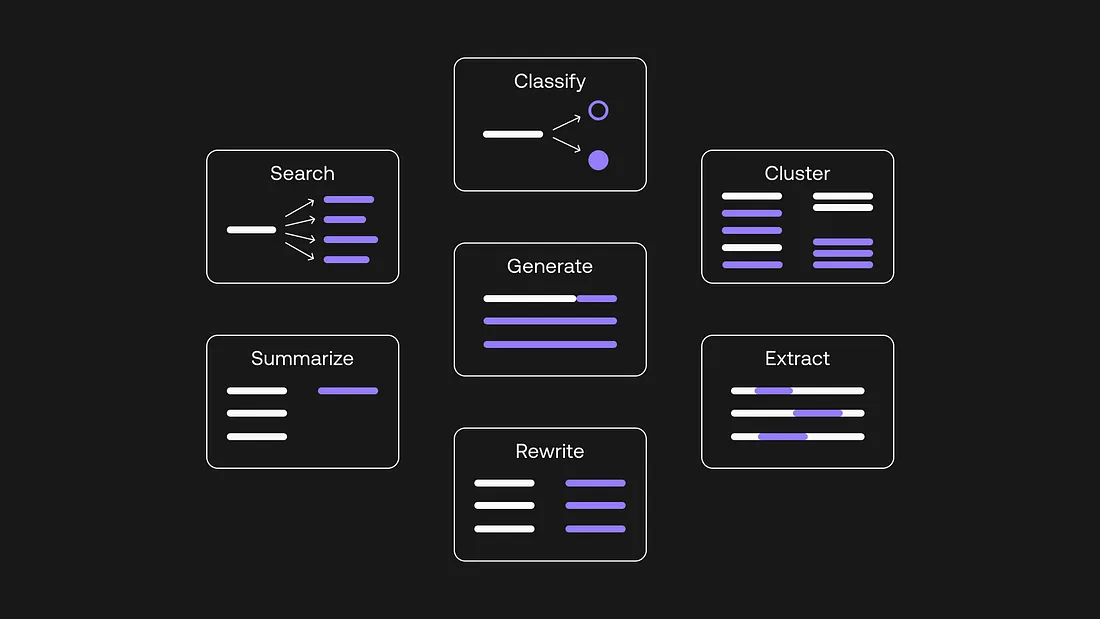
But Shipping LLMs to production is hard.
Creating a Proof of Concept use case with Large Language Models and OpenAI APIs is easy, but when you start thinking of production 🚀, a lot more considerations come into play.
For most companies, building the engineering capability to handle the complex GPU infra for serving LLMs reliably is difficult and time taking. Moreover, most companies want specific models that run best on their use case, for which they need to fine-tune these models. This can both be technically challenging and an expensive affair.

Ship open-source LLMs fast!
Our stance on the future of LLMs is that Open Source Models are going to be the way forward. Read more about our views on the topic here. We have decided to leverage this rapidly innovating community of innovators and help equip companies to utilize the complete value of these open-source LLMs in their organizations.
TrueFoundry wants that our partners can realize the full scale of advantages that Open-Source LLMs, fine-tuned for their specific use case, can have on their organizations:
- Cost-effective: 5-10X lower cost than using OpenAI APIs
- Complete Data Privacy: On your own cloud/on-prem Kubernetes Cluster
- Complete control: to fine-tune, rollback, etc
However, managing and deploying Open Source on one's own self is not an easy feat.
But imagine if it was just as easy as plugging in your data and a few clicks?
We understand the challenges businesses face when transitioning LLM proof of concept to production. We aim to build the layer that makes this process super easy for our partners. Here's how we do it:
Introducing the Model Catalogue
TrueFoundry's Model Catalogue is a repository of all the popular Open Source Large Language Models (LLMs) that can be deployed with a single click. The user can also fine-tune the model directly from the model catalogue.
The catalogue has most of the popular models already supported, and we are adding support for more every day. Some of the popular models that you could already deploy on your own cloud are:
- Pythia-70M
- Vicuna 7B 1.1 HF and Vicuna 13 B 1.1 HF
- Dolly V2 7B and Doly V2 12B
- Flan T5 XL
- Alpaca 7B and Alpaca 13B
And many more.....
The Magic we do on top of Open Source LLMs 🪄
We are obsessed that companies should be able to ship on day 1. To make this possible, here are the principles we are building our LLM capabilities on:
- Cost Optimization: Maximizes resource utilization to reduce infrastructure expenses without sacrificing performance.
- Simplified Deployment: Streamlined LLM deployment with containerization and Kubernetes for seamless scalability and high availability.
- Infrastructure Management: Handles intricate tasks like GPU allocation and Kubernetes management, freeing businesses to focus on LLM optimization.
- Pre-Built Abstractions: Provides ready-made solutions for easy integration of LLMs into existing workflows, eliminating the need for custom development.
- Support for Model Scaling: Enables scaling of LLMs of all sizes and types for optimal performance and efficiency.
ℹ️
For a detailed walkthrough of the training and fine-tuning flows on the UI refer to this YouTube video
Tutorial: Deploy LLMs within three clicks.
Deploying your LLMs is as easy as clicking three times!
- Select the Desired Model: Choose from a variety of open-source language models available on TrueFoundry. We suggest the best models for your use case. (Task-wise benchmarking is coming soon!) Select the model that best suits your specific problem or use case and click the deploy button.
- Choose the Appropriate Resources: Confirm the resources you want to allocate to the model. TrueFoundry offers a curated selection of hardware options optimized for each model to simplify the decision-making process that works well with the model you selected.
- Deploy the Model: Once you have selected the model and the deployment environment, simply click on the “Submit” button. TrueFoundry takes care of the behind-the-scenes tasks involved in setting up the infrastructure, configuring the model, and making it ready for inference.
🚀
Your model is now deployed!
Start Inference with the model API endpoint. TrueFoundry provides you with the OpenAPI interface to test your model and the sample code to call the model within your applications.

Tutorial: Fine-tuning Large Language Models with TrueFoundry

Most companies would want to use models fine-tuned for their specific use case. To fine-tune a model with TrueFoundry:
- Select the Desired Model: Choose the model you want to use from the catalog. Once you have selected the model, click on the "Fine-tune" button to initiate the process.
- Choose the Appropriate Resources: We pre-configure the suggested resources for the fine-tuning task. Users may change it if they anticipate a higher load due to changes in configs.
- Deploy the Finetuning Job: After selecting the model and the desired resources, click on the "Submit" button. TrueFoundry takes care of the behind-the-scenes tasks involved in setting up the infrastructure and configuring the training job. The finetuning job will start running, utilizing the specified hardware resources.
🚀
The model has started to fine-tune!
You can monitor the fine-tuning as it progresses. In the job runs tab, you can view all the relevant information associated with the training job, such as loss metrics, training curves, and evaluation results. This allows you to keep track of the finetuning process and make informed decisions based on the job's performance.
What is next?
This is only the start of our journey with Large Language Models (LLMs) and Generative AI. We are planning to build much more in the days to come and would keep you guys posted!
Chat with us
We are still learning about this topic, as everyone else. In case you are trying to make use of Large Language Models in your organization, we would love to chat and exchange notes.