We are back with another episode of True ML Talks. In this, we again dive deep into MLOps and LLMs Applications at GitLab and we are speaking with Monmayuri Ray.
Monmayuri leads the AI research vertical at GitLab with a lot of focus on the LLMs over the last one year. And prior to that, she was an engineering manager in the ModelOps Division at GitLab. She also worked with other companies like Microsoft, eBay.
📌
Our conversations with Monmayuri will cover below aspects:
- ML and LLM use cases at GitLab
- GitLab's ML infrastructure evolution to support large language models (LLMs)
- GitLab's Journey with LLMs: From Open Source to Fine-Tuning
- Training Large Language Models at GitLab
- Triton vs. PyTorch, Ensembled GPUs, and Dynamic Batching for LLM Inference
- Challenges and Research in Evaluating LLMs at GitLab
- GitLab's LLM Architecture and the Future of LLMs
Watch the full episode below:
ML and LLM use cases @ GitLab
Machine learning (ML) is transforming the software development lifecycle, and GitLab is at the forefront of this innovation. GitLab is using ML to empower developers throughout their journey, from creating issues to merging requests to deploying apps.
One of the most exciting use cases for ML at GitLab is large language models (LLMs). GitLab is using LLMs and GenAI to develop new features for its products, such as code completion and issue summarization.
Benefits of ML for GitLab users
- Increased productivity: ML can help developers to be more productive by automating tasks such as code completion and issue summarization.
- Improved code quality: ML can help developers to write better code by identifying potential errors and suggesting improvements.
- Reduced development time: ML can help developers to reduce the time it takes to develop software by automating tasks and helping them to identify and fix problems more quickly.
- Improved developer experience: ML can help to improve the developer experience by making it easier to use GitLab products and by providing support and guidance.
How GitLab's ML infrastructure evolution to support large language models (LLMs)
GitLab has been at the forefront of using large language models (LLMs) to empower developers. As a result, GitLab has had to evolve its ML infrastructure to support these complex models.
Challenges
- Size and complexity: LLMs are much larger and more complex than traditional ML models, which means that they require more powerful hardware and software to train and deploy.
- Integration: GitLab's products are written in Ruby on Rails and JavaScript. This means that GitLab has had to find ways to integrate its ML infrastructure with these technologies.
- Distributed infrastructure: GitLab's ML infrastructure is distributed across multiple different cloud providers. This means that GitLab has had to develop ways to manage its ML infrastructure in a consistent and efficient way.
Solutions
To address the challenges mentioned above, GitLab has made a number of changes to its ML infrastructure. These changes can be categorized into the following areas:
- Hardware: GitLab has invested in new hardware, such as GPUs and TPUs, to support the training and deployment of LLMs.
- Software: GitLab has developed new training and deployment pipelines for LLMs. GitLab has also developed a number of integration solutions to allow its ML infrastructure to work with its Ruby on Rails and JavaScript applications.
- Management: GitLab has developed a number of tools and processes to help manage its distributed ML infrastructure.
GitLab's Journey with LLMs: From Open Source to Fine-Tuning
GitLab has been at the forefront of using large language models (LLMs) to empower developers. In the early days, GitLab started by using open source LLMs, such as Salesforce code gen. However, as the landscape has changed and LLMs have become more powerful, GitLab has shifted to fine-tuning its own LLMs for specific use cases, such as code generation.
Fine-tuning LLMs requires a significant investment in infrastructure, as these models are very large and complex. GitLab has had to develop new training and deployment pipelines for LLMs, as well as new ways to manage its ML infrastructure in a distributed environment.
One of the key challenges that GitLab has faced in fine-tuning LLMs is finding the right balance between cost and latency. LLMs can be very expensive to train and deploy, and they can also be slow to generate results. GitLab has had to experiment with different cluster sizes, GPU configurations, and batching techniques to find the right balance for its needs.
Another challenge that GitLab has faced is ensuring that its LLMs are accurate and reliable. LLMs can be trained on massive datasets of text and code, but these datasets can also contain errors and biases. GitLab has had to develop new techniques to evaluate and debias its LLMs.
Despite the challenges, GitLab has made significant progress in using LLMs to empower developers. GitLab is now able to train and deploy LLMs at scale, and it is using these models to develop new features and products that will make the software development process more efficient and enjoyable.
Training Large Language Models at GitLab
Training large language models (LLMs) is a challenging task that requires a significant investment in infrastructure and resources. GitLab has been at the forefront of using LLMs to empower developers, and the company has learned a lot along the way.
Here are some insights and lessons learned from GitLab's experience training LLMs:
- Start small and scale up. When estimating the amount of GPU resources needed for training, it is best to start small and scale up gradually. This will help you to avoid wasting resources and to identify any potential bottlenecks early on.
- Use dynamic batching. Dynamic batching can help you to optimize your training process by grouping similar inputs together. This can lead to significant performance improvements, especially for large datasets.
- Choose the right parameters to optimize for. There is no one-size-fits-all approach to choosing the right parameters to optimize for when fine-tuning LLMs. The best parameters will vary depending on the specific LLM, training data, and desired outcome. However, it is important to experiment with different parameters to find the best combination for your specific needs.
- Consider using distributed training. Distributed training can help you to speed up the training process by distributing the workload across multiple GPUs or machines. This can be especially beneficial for training large LLMs on large datasets.
- Experiment with low rank adaptation mode. Low rank adaptation mode is a technique that can be used to fine-tune LLMs with a smaller number of parameters. This can be useful for cases where you do not have enough resources to fine-tune the entire model.
In addition to the above insights, GitLab has also learned a number of valuable lessons about the importance of having a good understanding of the base model and the training data. For example, GitLab has found that it is important to know the construct of the base model and how to curate the training data to optimize for the desired use case.
Triton vs. PyTorch, Ensembled GPUs, and Dynamic Batching for LLM Inference
GitLab uses Triton for LLM inference because it is better suited for scaling to the high volume of requests that GitLab receives. Triton is also easier to wrap and scale than other model servers, such as PyTorch servers.
GitLab has not yet experimented with Hugging Face's TGI or VLLM model servers, as these were still in the early stages of development when GitLab first deployed its LLM inference pipeline.
When it comes to dynamic batching, GitLab's strategy is to optimize for the specific use case, load, query level, volume, and number of GPUs available. For example, if GitLab has 500 GPUs for a 7B model, it can use a different batching strategy than if it only has a few GPUs for a smaller model.
GitLab also uses an ensemble of GPUs to handle requests. This means that GitLab uses a mix of different types of GPUs, including high-performance GPUs and lower-performance GPUs. GitLab load balances requests across the ensemble of GPUs to optimize for performance and cost.
Here are some tips for designing an architecture to ensemble GPUs and optimize load balancing:
- Understand your traffic patterns. When are your peak traffic times? What types of requests do you receive most often?
- Use A/B testing to experiment with different GPU configurations and load balancing strategies.
- Monitor your performance and latency to ensure that your architecture is meeting your needs.
Here are some specific examples of how GitLab has optimized its architecture for ensembled GPUs and dynamic batching:
- GitLab uses a dynamic orchestration system to assign requests to GPUs based on their type, performance, and availability.
- GitLab uses a technique called "GPU warm-up" to ensure that GPUs are ready to handle requests when they are needed.
- GitLab uses quantization to reduce the size of its models without sacrificing accuracy.
By following these tips, you can design an architecture that can efficiently handle large volumes of LLM inference requests.
We have tried streaming as well, and I think we are looking into streamings for our third parties as well - Monmayuri
Challenges and Research in Evaluating LLMs at GitLab
Evaluating the performance of large language models (LLMs) is a challenging task. GitLab has been working on this problem and has faced several challenges, including:
- Different use cases have different needs. Different LLM use cases, such as chat, code suggestion, and vulnerability explanation, have different needs and require different evaluation metrics.
- It is difficult to know which model works best for a given query. It is difficult to determine which LLM will perform best on a given query, especially in production.
- It is difficult to balance accuracy and acceptance rates. It is important to find a balance between the accuracy of the LLM's results and the acceptance rate of these results by users.
GitLab is addressing these challenges by:
- Curating a good dataset for each use case. GitLab is curating a dataset for each LLM use case that is representative of the types of queries that users will submit in production.
- Analyzing historic data. GitLab is analyzing historic data to understand how LLMs have performed on different types of queries in the past.
- Developing new evaluation metrics. GitLab is developing new evaluation metrics that are tailored to specific LLM use cases.
- Using data to drive decision-making. GitLab is using data to make decisions about which LLMs to use for different use cases and how to tune the parameters of these LLMs.
GitLab's goal is to develop a scalable and data-driven approach to evaluating LLMs. This approach will help GitLab to ensure that its LLMs are performing well in production and meeting the needs of its users.
Research directions
GitLab is also conducting research on new ways to evaluate LLMs. Some of the research directions that GitLab is exploring include:
- Using human-computer interaction (HCI) data to evaluate LLMs. HCI data can provide insights into how users interact with LLMs and how they perceive the results of these interactions. This data can be used to develop new evaluation metrics for LLMs.
- Using adversarial methods to evaluate LLMs. Adversarial methods can be used to generate inputs that are designed to trick LLMs into making mistakes. This data can be used to evaluate the robustness of LLMs to different types of errors.
- Using transfer learning to evaluate LLMs. Transfer learning can be used to evaluate LLMs on new tasks without having to collect a new dataset for each task. This can be useful for evaluating LLMs on tasks that are difficult or expensive to collect data for.
GitLab's research on evaluating LLMs is ongoing. GitLab is committed to developing new and innovative ways to evaluate LLMs so that it can ensure that its LLMs are meeting the needs of its users.
GitLab's LLM Architecture and the Future of LLMs
GitLab's LLM architecture is a comprehensive approach to training, evaluating, and deploying LLMs. The architecture is designed to be flexible and scalable, so that GitLab can easily adopt new technologies and meet the needs of its users.
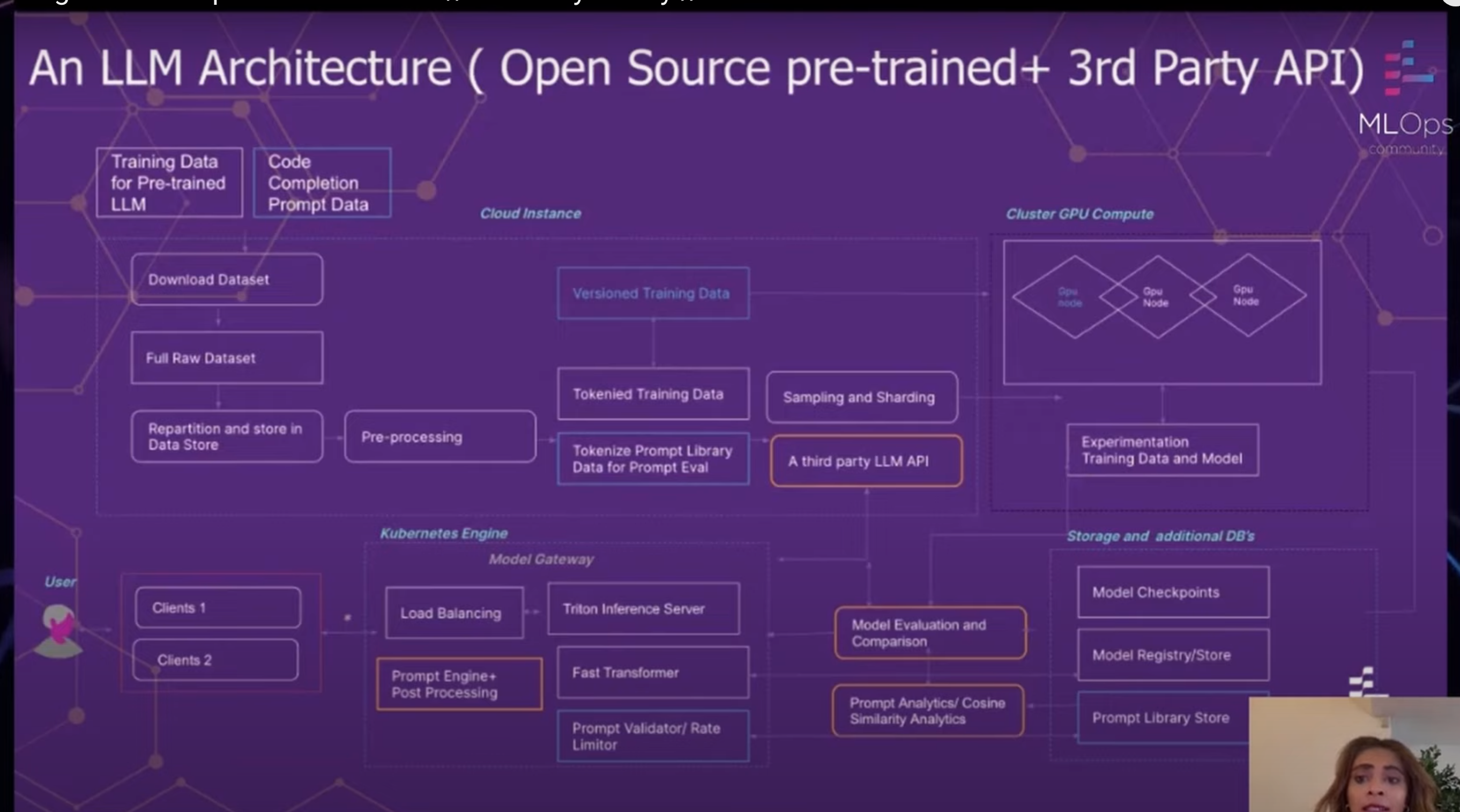
The architecture consists of several key components:
- Data preprocessing and tokenization: GitLab's LLM architecture starts by preprocessing and tokenizing the data that will be used to train the LLM. This process involves cleaning the data, removing noise, and converting the text into a format that the LLM can understand.
- Sampling and shortening: Once the data has been preprocessed and tokenized, GitLab's LLM architecture samples and shortens the data. This is done to reduce the computational cost of training the LLM.
- GPU suites: GitLab's LLM architecture uses GPU suites to train the LLM. GPUs are specialized processors that are well-suited for training LLMs.
- Evaluation suite: GitLab's LLM architecture includes an evaluation suite to evaluate the performance of the LLM. The evaluation suite includes a variety of metrics, such as accuracy, fluency, and coherence.
- Model checkpoints: GitLab's LLM architecture stores model checkpoints at regular intervals. This allows GitLab to resume training from a previous point if something goes wrong.
- Prompt library: GitLab's LLM architecture includes a prompt library, which is a collection of prompts that can be used to generate different types of text from the LLM.
- Model registry: GitLab's LLM architecture includes a model registry, which is a central repository for all of GitLab's LLM models.
- Deployment engine: GitLab's LLM architecture includes a deployment engine that deploys the LLM to production. The deployment engine includes a load balancer to distribute traffic across multiple instances of the LLM.
GitLab's LLM architecture is a powerful tool that enables GitLab to train, evaluate, and deploy LLMs at scale. The architecture is designed to be flexible and scalable, so that GitLab can easily adopt new technologies and meet the needs of its users.
The future of LLMs
LLMs are still a relatively new technology, but they have the potential to revolutionize many industries. GitLab believes that LLMs will have a significant impact on the software development industry.
GitLab is already using LLMs to improve its products and services. For example, GitLab is using LLMs to generate code suggestions, explain vulnerabilities, and improve the user experience of its products.
GitLab believes that other organizations should also invest in LLMs. LLMs have the potential to improve productivity, efficiency, and quality in many industries.
Areas to invest in
GitLab recommends that organizations invest in the following areas to stay ahead of the curve in the LLM space:
- Infrastructure: LLMs require a significant investment in infrastructure. Organizations need to invest in GPUs, storage, and networking to support LLMs.
- Tools and technologies: There are a number of tools and technologies that can help organizations to train, evaluate, and deploy LLMs. Organizations should invest in the tools and technologies that are right for their needs.
- Talent: LLMs are a complex technology. Organizations need to invest in talent with the skills and knowledge to train, evaluate, and deploy LLMs.
By investing in these areas, organizations can stay ahead of the curve in the LLM space and reap the benefits of this powerful technology.
Read our previous blogs in the True ML Talks series:
Keep watching the TrueML youtube series and reading the TrueML blog series.
TrueFoundry is a ML Deployment PaaS over Kubernetes to speed up developer workflows while allowing them full flexibility in testing and deploying models while ensuring full security and control for the Infra team. Through our platform, we enable Machine learning Teams to deploy and monitor models in 15 minutes with 100% reliability, scalability, and the ability to roll back in seconds - allowing them to save cost and release Models to production faster, enabling real business value realisation.